🌟 各位看官好,我是egoist2023!
🌍 种一棵树最好是十年前,其次是现在!
💬 注意:本文在哈希函数中主讲除法散列法,乘法散列法、全域散列法、双重散列等自行了解。
🚀 今天来学习哈希表的相关知识,为之后unordered_map/set的封装打下基础。
👍 如果觉得这篇文章有帮助,欢迎您一键三连,分享给更多人哦
引入 :直接定址法
在现实生活中,我们往往会将一类东西跟另一种东西进行绑定,且这种 关系具有一定的联系 。在计算机当中也是必然,如“left”的中文意思是“左边”,“string”的中文意思是“字符串”等等。而对于每个数字都有对应存储的下标。当 关键字的范围⽐较集中 时,⽐如⼀组关键字都在[0,99]之间,那么我们开⼀个100个数的数组,每个关键字的值直接就是存储位置的下标。但是如果 一组关键字比较分散 ,如只出现了1、20、99时,此时要开100空间的数组有97个空间会被浪费,这显然不是我们期望的。因此,关于一段 哈希的故事就此展开 。
哈希
哈希函数
除法散列法(除留余数法)
当数据比较分散的情况下,用直接定址法是无法很好地处理问题的,那是否能仅用较小地空间让保证所有的值都映射到该空间上来呢(保证空间大于值数量)?于是有人提出了除法散列法的概念并对此进行了说明。
除法散列法也叫做除留余数法,假设哈希表的大小为M,那么通过key除以M的余数作为映射位置的下标,也就是哈希函数为:h(key) = key % M。(这样即能保证所有的值都在这个空间上)
哈希冲突和负载因子
当使⽤除法散列法时,要 尽量避免M为某些值 ,如2的幂,10的幂等。如果是 ,那么key %2 ^X 本质相当于保留key的后X位,那么后x位相同的值,计算出的哈希值都是⼀样的,就冲突了。如:{63 , 31}看起来没有关联的值,如果M是16,即2^4,保留后4位,因为63的⼆进制后8位是 00111111,31的⼆进制后8位是 00011111,后四位都是相同的,那么都会映射到同一个空间上去,这样就产生了冲突,即哈希冲突。因此 当使⽤除法散列法时,建议M取不太接近2的整数次幂的⼀个质数(素数) 。负载因子:假设哈希表中已经映射存储了N个值,哈希表的⼤⼩为M,M一定要大于,那么负载因子 = N/M,保证负载因子小于1。负载因⼦越⼤,说明M是接近于N的,则空间利⽤率越⾼,相对地哈希冲突的概率越⾼;负载因⼦越⼩,说明M的空间很大,则空间利用率低,相对地哈希冲突的概率越低。
处理哈希冲突
开放定址法
enum Status
{
EMPTY,
EXIST,
DELETE
};
template<class K, class V>
struct HashData
{
pair<K, V> _kv;
Status _status = EMPTY;
};
template<class K, class V, class Hash = HashFunc<K>>
class HashTable
{
public:
HashTable(size_t size = 11)
:_tables(size)
, _n(0)
{}
//...
private:
vector<HashData<K, V>> _tables;
size_t _n;
};
优化
但是上面的实现并不是那么好,如果当映射的元素_n/_tables.size()大于负载因子时,显然是需要扩容的,如果选择2倍扩容,原本的11空间变为22空间,看似没有毛病。但前面我们说过,使用除法散列法时,要尽量避免M为某些值,即取不太接近2的整数次幂的⼀个质数。因此,不能直接地选择2倍扩容地方式来放大空间。那代码又该如何实现呢?
- 提前造好一些数据,这些数据得保证是质数,且是不太接近2的整数次幂的⼀个质数;
- 每次扩容的时候都往我们造好的数据上进行扩容。
static const int __stl_num_primes = 28;
static const unsigned long __stl_prime_list[__stl_num_primes] =
{
53, 97, 193, 389, 769,
1543, 3079, 6151, 12289, 24593,
49157, 98317, 196613, 393241, 786433,
1572869, 3145739, 6291469, 12582917, 25165843,
50331653, 100663319, 201326611, 402653189, 805306457,
1610612741, 3221225473, 4294967291
};
inline unsigned long __stl_next_prime(unsigned long n)
{
const unsigned long* first = __stl_prime_list;
const unsigned long* last = __stl_prime_list + __stl_num_primes;
const unsigned long* pos = lower_bound(first, last, n);
return pos == last ? *(last - 1) : *pos;
}
enum Status
{
EMPTY,
EXIST,
DELETE
};
template<class K, class V>
struct HashData
{
pair<K, V> _kv;
Status _status = EMPTY;
};
template<class K, class V, class Hash = HashFunc<K>>
class HashTable
{
public:
HashTable(size_t size = __stl_num_primes)
:_tables(size)
, _n(0)
{}
//...
private:
vector<HashData<K, V>> _tables;
size_t _n;
};
在上面这段程序中,哈希表默认初始空间是28,当大于负载因子时进行扩容,第一次扩容是53,第二次是97…… ,可以发现,每次扩容都是以近乎2倍扩容且满足不太接近2的整数次幂的⼀个质数。这种手动造数据的方法看似笨拙,实则这难道不是利用其特性下的一种取巧吗?
线性探测
- 在映射数据的时候可能会存在哈希冲突,此时从发⽣冲突的位置开始,依次线性向后探测,直到寻找到下⼀个没有存储数据的位置为⽌,如果⾛到哈希表尾,则回绕到哈希表头的位置。
- h(key) = hash0 = key % M,hash0位置冲突了,则线性探测公式为:hc(key,i) = hashi = (hash0 + i) % M, i = {1, 2, 3, ..., M − 1},保证线性探测时能从队尾走到队头,且因为负载因⼦小于1,则最多探测M-1次,⼀定能找到⼀个存储key的位置。
- 可以发现线性探测的问题会占用其他值可能映射到的空间,会导致原本不冲突的值产生哈希冲突。严重的话肯呢个会使多个hash0,hash1,hash2,hash3的值都争夺hash3位置,这种现象叫做群集/堆积。
二次探测(了解)
- 从发⽣冲突的位置开始,依次左右按⼆次⽅跳跃式探测,直到寻找到下⼀个没有存储数据的位置为止,如果往右⾛到哈希表尾,则回绕到哈希表头的位置;如果往左⾛到哈希表头,则回绕到哈希表尾的位置;
- h(key) = hash0 = key % M , hash0位置冲突了,则⼆次探测公式为:hc(key,i) = hashi = (hash0 ± i^2 ) % M, i = {1, 2, 3, ...,M/2 };
- ⼆次探测当 hashi = (hash0 − i 2 )%M 时,当hashi<0时,需要hashi += M。
线性探测实现
bool Insert(const pair<K, V>& kv)
{
size_t hash0 = kv.first % _tables.size();
size_t hashi = hash0;
size_t i = 1;
//如果该点存在 --> 线性探测
while (_tables[hashi]._status == EXIST)
{
hashi = (hashi + i) % _tables.size();
i++;
}
_tables[hash0]._kv = kv;
_tables[hash0]._status = EXIST;
++_n;
return true;
}
扩容
方案一:新建一个哈希表,遍历旧表让里面的数据重新映射到新表当中;
方案二:采用复用的手段将旧表数据映射到新表中。
if ((double)_n / (double)_tables.size() > 0.7)
{
HashTable<K, V, Hash> newHT(__stl_next_prime(_tables.size() + 1));
for (size_t i = 0;i < _tables.size();i++)
{
if (_tables[i]._status == EXIST)
{
newHT.Insert(_tables[i]._kv);
}
}
_tables.swap(newHT._tables);
}
查找和删除
查找规律:巧妙利用线性探测的特性
删除规律:采用Find函数寻找该值是否存在,如果存在标记为del,返回true;否则,返回false。
HashData<K, V>* Find(const K& key) { size_t hash0 = key % _tables.size(); size_t hash1 = hash0; size_t i = 1; while (_tables[hash1]._status != EMPTY) { if (_tables[hash1]._kv.first == key && _tables[hash1]._status != DELETE) { return &_tables[hash1]; } hash1 = (hash1 + i) % _tables.size(); ++i; } return nullptr; } bool Erase(const K& key) { HashData<K, V>* ret = Find(key); if (ret) { ret->_status = DELETE; return true; } else { return false; } }
代码实现
static const int __stl_num_primes = 28;
static const unsigned long __stl_prime_list[__stl_num_primes] =
{
53, 97, 193, 389, 769,
1543, 3079, 6151, 12289, 24593,
49157, 98317, 196613, 393241, 786433,
1572869, 3145739, 6291469, 12582917, 25165843,
50331653, 100663319, 201326611, 402653189, 805306457,
1610612741, 3221225473, 4294967291
};
inline unsigned long __stl_next_prime(unsigned long n)
{
const unsigned long* first = __stl_prime_list;
const unsigned long* last = __stl_prime_list + __stl_num_primes;
const unsigned long* pos = lower_bound(first, last, n);
return pos == last ? *(last - 1) : *pos;
}
enum Status
{
EMPTY,
EXIST,
DELETE
};
template<class K, class V>
struct HashData
{
pair<K, V> _kv;
Status _status = EMPTY;
};
template<class K>
struct HashFunc
{
size_t operator()(const K& key)
{
return (size_t)key;
}
};
template<class K, class V, class Hash = HashFunc<K>>
class HashTable
{
public:
HashTable(size_t size = __stl_num_primes)
:_tables(size)
, _n(0)
{}
bool Insert(const pair<K, V>& kv)
{
//扩容 --> 负载因子大于0.7
if ((double)_n / (double)_tables.size() > 0.7)
{
HashTable<K, V, Hash> newHT(__stl_next_prime(_tables.size() + 1));
for (size_t i = 0;i < _tables.size();i++)
{
if (_tables[i]._status == EXIST)
{
newHT.Insert(_tables[i]._kv);
}
}
_tables.swap(newHT._tables);
}
size_t hash0 = kv.first % _tables.size();
size_t hashi = hash0;
size_t i = 1;
//如果该点存在 --> 线性探测
while (_tables[hashi]._status == EXIST)
{
hashi = (hashi + i) % _tables.size();
i++;
}
_tables[hash0]._kv = kv;
_tables[hash0]._status = EXIST;
++_n;
return true;
}
HashData<K, V>* Find(const K& key)
{
size_t hash0 = key % _tables.size();
size_t hash1 = hash0;
size_t i = 1;
while (_tables[hash1]._status != EMPTY)
{
if (_tables[hash1]._kv.first == key && _tables[hash1]._status != DELETE)
{
return &_tables[hash1];
}
hash1 = (hash1 + i) % _tables.size();
++i;
}
return nullptr;
}
bool Erase(const K& key)
{
HashData<K, V>* ret = Find(key);
if (ret)
{
ret->_status = DELETE;
return true;
}
else
{
return false;
}
}
private:
vector<HashData<K, V>> _tables;
size_t _n;
};
链地址法
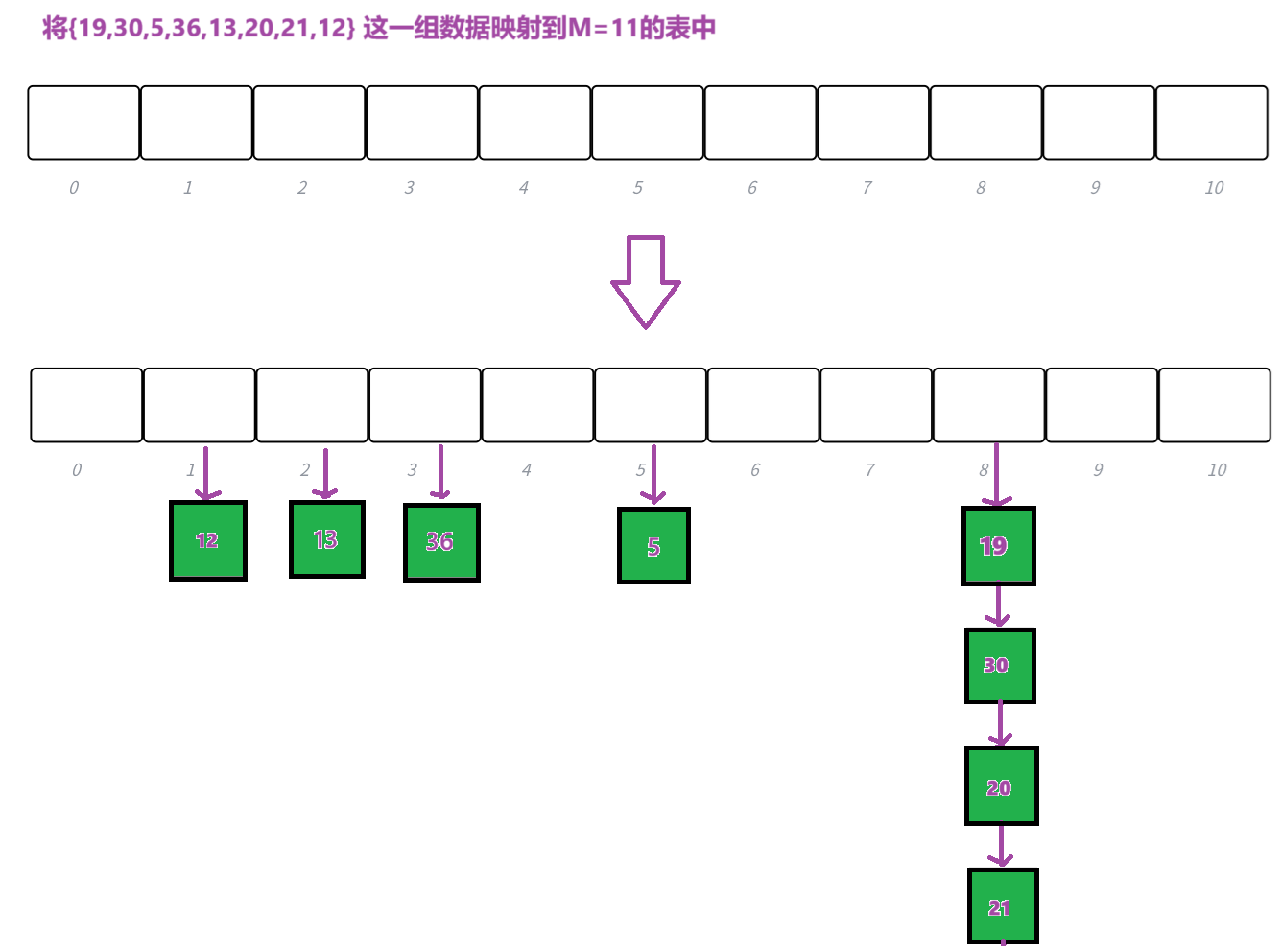
但是在一些极端场景下,如有人恶意造出一段数据让它都映射到同一个位置,导致某个桶特别长,查询时间复杂度达到了O(N)。有大佬提出,如果这个桶的长度超过⼀定阀值(8)时就把链表转换成红⿊树。(用全域散列法也可)
链地址法的结构实现
//手动造数据见上面
template<class K,class V>
struct HashNode
{
pair<K, V> _kv;
HashNode<K, V>* _next;
HashNode(const pair<K, V>& kv)
:_next(nullptr)
,_kv(kv)
{}
};
template<class K, class V>
class HashTable
{
typedef HashNode<K, V> Node;
public:
HashTable(size_t size = __stl_next_prime(0))
:_tables(size, nullptr)
,_n(0)
{}
private:
vector<Node*> _tables;
size_t _n;
};
特殊情况:插入元素不是数字
如果插入元素是浮点数、负数情况呢?
仿函数的作用再次体现出来了,无论是整数、浮点数、负数,统一强转成正数来处理,找到该映射的位置,对查找、删除等操作都不会受到影响,因为我们同样需要将目标值强制转成正数映射到对应位置。
//仿函数
template<class K>
struct HashFunc
{
size_t operator()(const K& key)
{
return (size_t)key; // --> 针对浮点、负数等情况
}
};
针对字符串
但是字符串的处理,并不能直接强转成正数来处理,这并不被允许。因此,在这里采用特化的思想进行特殊处理,实现如下。
//针对字符串
template<>
struct HashFunc<string>
{
size_t operator()(const string& key)
{
size_t hash0 = 0;
for (auto& ch : key)
{
//对不同字符串但hash0相同的处理,减少冲突
hash0 *= 131;
hash0 += ch;
}
return hash0;
}
};
改动
template<class K, class V, class Hash = HashFunc<K>>
扩容
- 方法一:由于是链地址法的实现,一个位置下可能会挂着多个值(即哈希桶),那么就需要遍历该位置中桶的每个结点,将每个结点的值重新映射到新表中,而每次操作都需要申请新结点,并把旧表中该结点释放掉。显然,这种方式是非常浪费的,且效率非常低效。
- 方法二:正是由于采用的是链地址法的实现,由于哈希表中每个位置都是一个指针,那么我们只需要遍历旧表中结点映射在新表中的位置,使其指向旧表中该结点。最后,将旧表置为空,交换新旧链表,完成扩容操作。
bool Insert(const pair<K, V>& kv)
{
//不允许冗余
if (Find(kv.first))
return false;
Hash hs;
//需要扩容
if (_n == _tables.size())
{
// 也可以,但是扩容新开辟节点,释放旧节点,有点浪费
/*HashTable<K, V> newHT(__stl_next_prime(_tables.size() + 1));
for (size_t i = 0; i < _tables.size(); i++)
{
Node* cur = _tables[i];
while (cur)
{
newHT.Insert(cur->_kv);
cur = cur->_next;
}
}
_tables.swap(newHT._tables);*/
vector<Node*> newtables(__stl_next_prime(_tables.size() + 1), nullptr);
//遍历旧表
for (size_t i = 0;i < _tables.size();i++)
{
Node* cur = _tables[i];
while (cur)
{
//将旧表的每个结点插在新表中
Node* next = cur->_next;
size_t hash0 = hs(cur->_kv.first) % newtables.size();
cur->_next = newtables[hash0];
newtables[hash0] = cur;
cur = next;
}
_tables[i] = nullptr;
}
_tables.swap(newtables);
}
size_t hash0 = hs(kv.first) % _tables.size();
Node* newnode = new Node(kv);
//头插
newnode->_next = _tables[hash0];
_tables[hash0] = newnode;
++_n;
return true;
}
删除
bool Erase(const K& key)
{
Hash hs;
size_t hash0 = hs(key) % _tables.size();
Node* prev = nullptr;
Node* cur = _tables[hash0];
while (cur)
{
if (cur->_kv.first == key)
{
if (prev == nullptr)
{
_tables[hash0] = cur->_next;
}
else
{
prev->_next = cur->_next;
}
--_n;
delete cur;
return true;
}
prev = cur;
cur = cur->_next;
}
return false;
}
代码实现
static const int __stl_num_primes = 28;
static const unsigned long __stl_prime_list[__stl_num_primes] =
{
53, 97, 193, 389, 769,
1543, 3079, 6151, 12289, 24593,
49157, 98317, 196613, 393241, 786433,
1572869, 3145739, 6291469, 12582917, 25165843,
50331653, 100663319, 201326611, 402653189, 805306457,
1610612741, 3221225473, 4294967291
};
inline unsigned long __stl_next_prime(unsigned long n)
{
const unsigned long* first = __stl_prime_list;
const unsigned long* last = __stl_prime_list + __stl_num_primes;
const unsigned long* pos = lower_bound(first, last, n);
return pos == last ? *(last - 1) : *pos;
}
template<class K,class V>
struct HashNode
{
pair<K, V> _kv;
HashNode<K, V>* _next;
HashNode(const pair<K, V>& kv)
:_next(nullptr)
,_kv(kv)
{}
};
//仿函数
template<class K>
struct HashFunc
{
size_t operator()(const K& key)
{
return (size_t)key; // --> 针对浮点、负数等情况
}
};
//针对字符串
template<>
struct HashFunc<string>
{
size_t operator()(const string& key)
{
size_t hash0 = 0;
for (auto& ch : key)
{
//对不同字符串但hash0相同的处理,减少冲突
hash0 *= 131;
hash0 += ch;
}
return hash0;
}
};
template<class K, class V, class Hash = HashFunc<K>>
class HashTable
{
typedef HashNode<K, V> Node;
public:
HashTable(size_t size = __stl_next_prime(0))
:_tables(size, nullptr)
,_n(0)
{}
bool Insert(const pair<K, V>& kv)
{
//不允许冗余
if (Find(kv.first))
return false;
Hash hs;
//需要扩容
if (_n == _tables.size())
{
// 也可以,但是扩容新开辟节点,释放旧节点,有点浪费
/*HashTable<K, V> newHT(__stl_next_prime(_tables.size() + 1));
for (size_t i = 0; i < _tables.size(); i++)
{
Node* cur = _tables[i];
while (cur)
{
newHT.Insert(cur->_kv);
cur = cur->_next;
}
}
_tables.swap(newHT._tables);*/
vector<Node*> newtables(__stl_next_prime(_tables.size() + 1), nullptr);
//遍历旧表
for (size_t i = 0;i < _tables.size();i++)
{
Node* cur = _tables[i];
while (cur)
{
//将旧表的每个结点插在新表中
Node* next = cur->_next;
size_t hash0 = hs(cur->_kv.first) % newtables.size();
cur->_next = newtables[hash0];
newtables[hash0] = cur;
cur = next;
}
_tables[i] = nullptr;
}
_tables.swap(newtables);
}
size_t hash0 = hs(kv.first) % _tables.size();
Node* newnode = new Node(kv);
//头插
newnode->_next = _tables[hash0];
_tables[hash0] = newnode;
++_n;
return true;
}
Node* Find(const K& key)
{
Hash hs;
size_t hashi = hs(key) % _tables.size();
Node* cur = _tables[hashi];
while (cur)
{
if (cur->_kv.first == key)
return cur;
cur = cur->_next;
}
return nullptr;
}
bool Erase(const K& key)
{
Hash hs;
size_t hash0 = hs(key) % _tables.size();
Node* prev = nullptr;
Node* cur = _tables[hash0];
while (cur)
{
if (cur->_kv.first == key)
{
if (prev == nullptr)
{
_tables[hash0] = cur->_next;
}
else
{
prev->_next = cur->_next;
}
--_n;
delete cur;
return true;
}
prev = cur;
cur = cur->_next;
}
return false;
}
~HashTable()
{
for (size_t i = 0;i < _tables.size();i++)
{
Node* cur = _tables[i];
while (cur)
{
Node* next = cur->_next;
delete cur;
cur = next;
}
_tables[i] = nullptr;
}
}
private:
vector<Node*> _tables;
size_t _n;
};